Stochastic Image Spectroscopy - SIS
Stochastic Image Spectroscopy (SIS) has been a primary line of research within our laboratory for years. It is a novel, model-based strategy for hyperspectral image analysis and classification, that does not rely on spectral feature descriptions or strong pixel-level discriminating assumptions. It employs a hierarchical Bayesian generative imaging model that incorporates several hidden layers of information, offering descriptions of hyperspectral images at a higher semantic level of abstraction called model-based hyperspectral identity signature. This novel method has consistently delivered highly promising results.
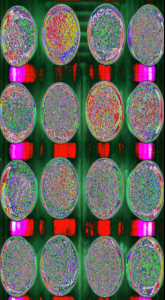
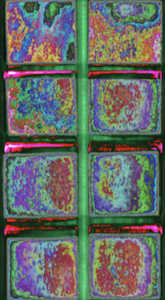
Initial Approach
The initial paper from this study (Egaña et al., 2020) addressed a major challenge in hyperspectral analysis: the inherent variability of the sampling process, where achieving ideal acquisition conditions, free from external influences, is nearly impossible. Traditional image classification methods, which rely on a feature-based discrimination hypothesis, often overlook the stochastic nature of hyperspectral image acquisition, leading to limitations when facing unideal sampling conditions.
Conversely, the approach presented in (Egaña et al., 2020) focuses on the characterization and analysis of the spectral and spatial variability of the surface under study. The proposed framework is broadly composed of:
(a) a new hyperspectral image segmentation algorithm - which allows for the combination of spectral and spatial information
(b) a preserving-information dimensionality reduction scheme - which improves processing time and overall performance
(c) a stochastic hierarchical regression model - for characterizing the inherent variability of spectra

Testing
In line with this research, a hyperspectral dataset was constructed for its use in regression and classification studies, as presented in (Ehrenfeld et al., 2023).
The dataset, derived from five distinct sets of geometallurgical samples, was captured using multipixel hyperspectral imagenology, spanning wavelengths from 400 to 2500 nm. Thousands of spectra pixels were captured from each mineral sample, providing statistically representative spectral information, suitable for a wide range of analyses beyond geometallurgical studies.
For technical validation, the dataset was tested across various regression and classification experiments, employing the methodology proposed in (Egaña et al., 2020).
Current Work
SIS has evolved significantly over its extensive trajectory. Through numerous tests and collaborations with various industries, it has transitioned from an initial implementation pipeline into a model that provides a robust framework for analyzing and classifying hyperspectral images, effective even in the absence of distinct spectral components. Moreover, training the model does not require expensive pixel-level data, and it is resilent against occlusion, missing spectral observations, and poor spectral sensing conditions.
The framework has been formalized into a software librery named HyBEL, which offers state-of-the-art results for many hyperspectral image classification tasks.
The formalisation of the theory behind the generative SIS model is already published in the prestigious Springer-Nature journal Scientific Reports (Egaña et al., 2024).
References
Journal Articles
- [1]Stochastic image spectroscopy: a discriminative generative approach to hyperspectral image modelling and classification.In Scientific Reports, vol. 14, no. 1, p. 19308, 2024.
- [2]HIDSAG: Hyperspectral Image Database for Supervised Analysis in Geometallurgy.In Scientific Data, vol. 10, no. 1, p. 164, Mar. 2023.
- [3]A robust stochastic approach to mineral hyperspectral analysis for geometallurgy.In Minerals, vol. 10, no. 12, pp. 1–32, 2020.